Publications
Google Scholar Page for a list of my most recent preprints.
D. Klein, G. Palla, M. Lange, M. Klein, Z. Piran, M. Gander, L. Meng-Papaxanthos, M. Sterr, L. Saber, C. Jing, A. Bastidas-Ponce, P. Cota, M. Tarquis-Medina, S. Parikh, I. Gold, H. Lickert, M. Bakhti, M. Nitzan, MC, F. Theis, Mapping cells through time and space with moscot, Nature
P. Rodriguez, A. Blaas, M. Klein, L. Zappella, N. Apostoloff, MC, Xavier Suau, Controlling Language and Diffusion Models by Transporting Activations, ICLR 2025
T. Uscidda, L. Eyring, K. Roth, F. Theis, Z. Akata, MC, Disentangled Representation Learning with the Gromov-Monge Gap, ICLR 2025
B. Kim, Y.-G. Hsieh, M. Klein, MC, J.-C. Ye, B. Kawar, J. Thornton, Simple ReFlow: Improved Techniques for Fast Flow Models, ICLR 2025
P. Kassraie, AA. Pooladian, M. Klein, J. Thornton, J. Niles-Weed, MC, Progressive Entropic Optimal Transport Solvers, NeurIPS 2024
M. Klein, AA. Pooladian, P. Ablin, E. Ndiaye, J. Niles-Weed, MC, Learning Elastic Costs to Shape Monge Displacements, NeurIPS 2024
D. Klein, T. Uscidda, F. Theis, MC, Entropic Gromov Wasserstein Flow Matching, NeurIPS 2024
C. Bunne, G. Schiebinger, A. Krause, A. Regev, MC, Optimal transport for single-cell and spatial omics, Nature Reviews Methods Primers volume 4, 58, 2024
Z. Piran, M. Klein, J. Thornton, MC, Contrasting Multiple Representations with the Multi-Marginal Matching Gap (oral), ICML 2024
YG. Hsieh, J. Thornton, E. Ndiaye, M. Klein, MC, P. Ablin, Careful with that Scalpel: Improving Gradient Surgery with an EMA, ICML 2024
N. Vesseron, MC, On a Neural Implementation of Brenier's Polar Factorization, ICML 2024
T. Lin, MC, M. Jordan, A Specialized Semismooth Newton Method for Kernel-Based Optimal Transport, AISTATS 2024
O. Sebbouh, MC, G. Peyré, Structured Transforms Across Spaces with Cost-Regularized Optimal Transport, AISTATS 2024
M. Scetbon, M. Klein, G. Palla, MC, Unbalanced Low-Rank Optimal Transport Solvers, NeurIPS 2023
MC, M. Klein, P. Ablin, Monge, Bregman and Occam: Interpretable Optimal Transport in High-Dimensions with Feature-Sparse Maps, ICML 2023
T. Uscidda, MC, The Monge Gap: A Regularizer to Learn All Transport Maps, ICML 2023
J. Thornton, MC, Rethinking Initialization of the Sinkhorn Algorithm, AISTATS 2023
C. Bunne, YP. Hsieh, MC, A. Krause, Recovering Stochastic Dynamics via Gaussian Schrödinger Bridges, AISTATS 2023
C. Bunne, A. Krause, MC, Supervised Training of Conditional Monge Maps, NeurIPS 2022
M. Scetbon, MC, Low-Rank Optimal Transport: Approximation, Statistics and Debiasing, NeurIPS 2022
M. Blondel, Q. Berthet, MC, R. Frostig, S. Hoyer, F. Llinares-López, F. Pedregosa, J.-P. Vert Efficient and Modular Implicit Differentiation, NeurIPS 2022
M. Scetbon, G. Peyré, MC, Linear-Time Gromov Wasserstein Distances using Low Rank Couplings and Costs, ICML 2022
A.-A. Pooladian, MC, J. Niles-Weed, Debiaser Beware: Pitfalls of Centering Regularized Transport Maps, ICML 2022
T. Lin, N. Ho, MC, M. Jordan, On the Complexity of Approximating Multimarginal Optimal Transport, JMLR (23) 65
Y. Tian, MC, D. Ha, Simultaneous Multiple-Prompt Guided Generation Using Differentiable Optimal Transport, ICCC 2022
C. Bunne, L. Meng-Papaxanthos, A. Krause, MC, Proximal Optimal Transport Modeling of Population Dynamics, AISTATS 2022
O. Sebbouh, MC, G. Peyré, Randomized Stochastic Gradient Descent Ascent, AISTATS 2022
M. Scetbon, MC, G. Peyré, Low-Rank Sinkhorn Factorization, ICML 2021
Tianyi Lin, Zeyu Zheng, Elynn Y. Chen, MC, Michael I. Jordan, On Projection Robust Optimal Transport: Sample Complexity and Model Misspecification, AISTATS 2021
M. Scetbon, L. Meunier, J. Atif, MC, Equitable and Optimal Transport with Multiple Agents, AISTATS 2021
M. Heitz, N. Bonneel, D. Coeurjolly, MC, G. Peyré, Ground Metric Learning on Graphs, Journal of Mathematical Imaging and Vision, 1-19
T. Lin, C. Fan, N. Ho, MC, M.I. Jordan, Projection Robust Wasserstein Distance and Riemannian Optimization, NeurIPS 2020
M. Scetbon, MC, Linear Time Sinkhorn Divergences using Positive Features, NeurIPS 2020
H. Janati, B. Muzellec, G. Peyré, MC, Entropic Optimal Transport between Unbalanced Gaussian Measures has a Closed Form, NeurIPS 2020 (oral)
Q. Berthet, M. Blondel, O. Teboul, MC, J.-P. Vert, F. Bach, Learning with differentiable perturbed optimizers, NeurIPS 2020
T. Lin, N. Ho, X. Chen, MC, M.I. Jordan, Fixed-Support Wasserstein Barycenters:Computational Hardness and Fast Algorithm, NeurIPS 2020
H. Janati, T. Bazeille, B. Thirion, MC, A. Gramfort, Multi-subject MEG/EEG source imaging with sparse multi-task regression, Neuroimage, 2020
B. Muzellec, J. Josse, C. Boyer, MC, Missing Data Imputation using Optimal Transport, ICML 2020
H. Janati, MC, A. Gramfort, Debiased Sinkhorn Barycenters, ICML 2020
MC, O. Teboul, J. Niles-Weed, J.-P. Vert, Supervised Quantile Normalization for Low Rank Matrix Factorization, ICML 2020
F.P. Paty, MC, Regularized Optimal Transport is Ground Cost Adversarial, ICML 2020
F.P. Paty, A. d'Aspremont, MC, Regularity as regularization: Smooth and strongly convex brenier potentials in optimal transport, AISTATS 2020 (Notable paper award)
H. Janati, MC, A. Gramfort, Spatio-Temporal Alignments: Optimal transport through space and time, AISTATS 2020.
J. Djolonga, M. Lucic, MC, O. Bachem, O. Bousquet, Sylvain Gelly Evaluating Generative Models Using Divergence Frontiers, AISTATS 2020.
MC, O. Teboul, J.P. Vert, Differentiable Sorting using Optimal Transport, Neurips 2019.
B. Muzellec, MC, Subspace Detours: Building Transport Plans that are Optimal on Subspace Projections, Neurips 2019.
T. Le, M. Yamada, K. Fukumizu, MC, Tree-Sliced Variants of Wasserstein Distances, Neurips 2019.
F.P. Paty, MC, Subspace Robust Wasserstein Distances, ICML 2019.
G. de Bie, G. Peyré, MC, Stochastic Deep Networks, ICML 2019.
H. Janati, T. Bazeille, B. Thirion, MC, A. Gramfort, Group level MEG/EEG source imaging via optimal transport: minimum Wasserstein estimates, IPMI, 2019.
G. Peyré, MC, Computational Optimal Transport, FnT in Machine Learning, 11(5-6), 2019.
S.-I. Amari, R. Karakida, M. Oizumi, MC, Information Geometry for Regularized Optimal Transport and Barycenters of Patterns, Neural Computation, 2019.
A. Genevay, L. Chizat, F. Bach, MC, G. Peyré, Sample Complexity of Sinkhorn Divergences, AISTATS 2019.
H. Janati, MC, A. Gramfort, Wasserstein Regularization for Sparse Multi-task Regression, AISTATS 2019.
J. Alaux, E. Grave, MC, A. Joulin Unsupervised Hyper-alignment for Multilingual Word Embeddings, ICLR 2019.
MC, G. Peyré, Semidual Regularized Optimal Transport, SIAM Review, 2018
T. Lacombe, MC, S. Oudot, Large Scale computation of Means and Clusters for Persistence Diagrams using Optimal Transport, NeurIPS 2018.
B. Muzellec, MC,
Generalizing Point Embeddings using the Wasserstein Space of Elliptical Distributions, NeurIPS 2018.
R. Flamary, MC, N. Courty, A. Rakotomamonjy, Wasserstein Discriminant Analysis, Machine Learning Journal, 2018.
E. Cazelles, V. Seguy, J. Bigot, MC, N. Papadakis, Log-PCA versus Geodesic PCA of histograms in the Wasserstein space, to appear in SIAM J. on Scient. Comput., 2018
M.A. Schmitz, M. Heitz, N. Bonneel, F.M. Ngolè Mboula, D. Coeurjolly, MC, G. Peyré, J.-L. Starck, Wasserstein Dictionary Learning: Optimal Transport-based unsupervised non-linear dictionary learning, SIAM J. Imaging Sci. (11-1), 2018
A. Genevay, G. Peyré, MC, Learning Generative Models with Sinkhorn Divergences, AISTATS 2018.
M. Carrière, MC, S. Oudot, Sliced Wasserstein Kernel for Persistence Diagrams, ICML 2017.
A. Ramdas, N. García Trillos, MC, On Wasserstein Two-Sample Testing and Related Families of Nonparametric Tests, Entropy 19(2), 2017
A. Genevay, MC, G. Peyré, F. Bach, Stochastic Optimization for Large-scale Optimal Transport, NIPS 2016.
G. Montavon, K.-R. Müller, MC, Wasserstein Training of Restricted Boltzmann Machines, NIPS 2016.
G. Peyré, MC, J. Solomon, Gromov-Wasserstein Averaging of Kernel and Distance Matrices, ICML 2016.
N. Bonneel, G. Peyré, MC, Wasserstein Barycentric Coordinates: Histogram Regression Using Optimal Transport, SIGGRAPH 2016.
A. Rolet, MC, G. Peyré, Fast Dictionary Learning with a Smoothed Wasserstein Loss, AISTATS 2016.
MC, G. Peyré, A Smoothed Dual Formulation for Variational Wasserstein Problems, SIAM J. Imaging Sci. (9-1), 2016.
V. Seguy, MC, Principal Geodesic Analysis for Probability Measures under the Optimal Transport Metric, NIPS 2015.
J. Solomon, F. de Goes, G. Peyré, MC, A. Butscher, A. Nguyen, T. Du, L. Guibas, Convolutional Wasserstein Distances: Efficient Optimal Transportation on Geometric Domains, SIGGRAPH 2015.
J.D. Benamou, G. Carlier, MC, L. Nenna, G. Peyré, Iterative Bregman Projections for Regularized Transportation Problems, SIAM J. on Scient. Comput.(37-2), 2015.
A. Gramfort, G. Peyré, MC, Fast Optimal Transport Averaging of Neuroimaging Data, IPMI 2015.
MC, A. Doucet, Fast Computation of Wasserstein Barycenters, ICML 2014. 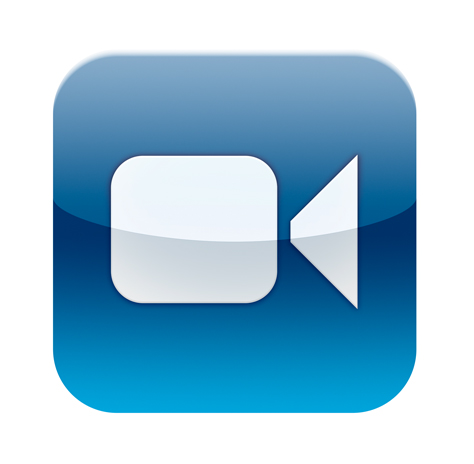
MC, D. Avis, Ground Metric Learning, JMLR, 2014.
MC, Sinkhorn Distances: Lightspeed Computation of Optimal Transport, NIPS 2013. 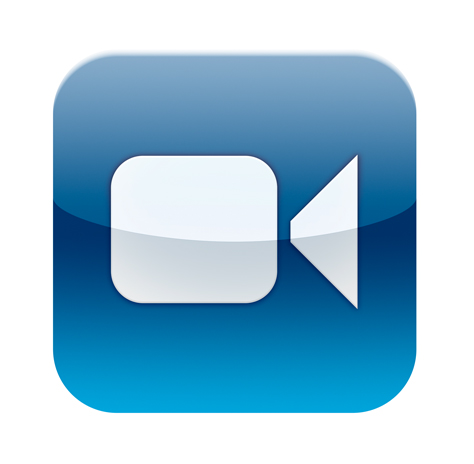
MC, Permanents, Transportation Polytopes and Positive Definite Kernels on Histograms, IJCAI 2007. Note: the proof of Prop.3 in that paper is wrong. See this paper for a correct result.
Time Series
MC, M. Blondel, Soft-DTW: A Differentiable Loss Function for Time Series, ICML 2017.
MC, A. d'Aspremont, Mean-Reverting Portfolios: Tradeoffs Between Sparsity and Volatility, Financial Signal Processing and Machine Learning, Wiley, 2016.
MC, A. d'Aspremont, Mean Reversion with a Variance Threshold, ICML 2013. 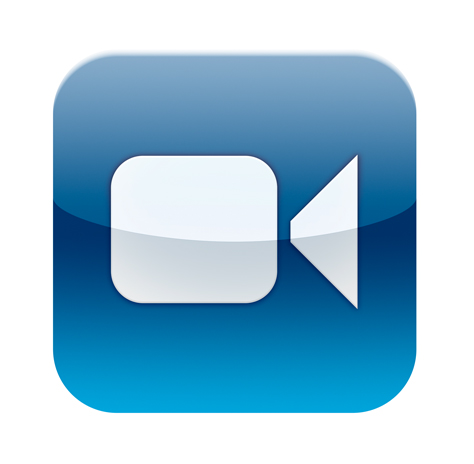
MC, Fast Global Alignment Kernels, ICML 2011. 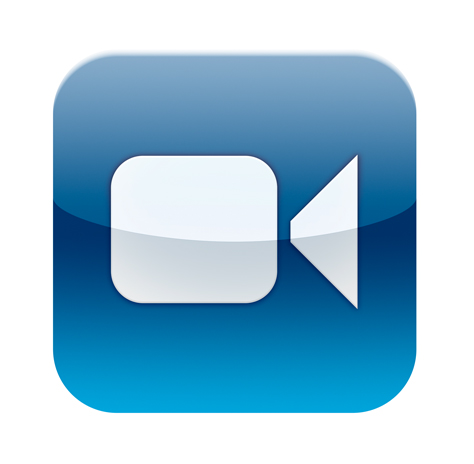
MC , J.-P. Vert, O. Birkenes, T. Matsui, A Kernel for Time Series based on Global Alignments, ICASSP 2007. 
Kernels / Metrics for Structured Data
T. Le, MC, Unsupervised Riemannian Metric Learning for Histograms Using Aitchison Transformations, ICML 2015.
T. Le, MC, Adaptive Euclidean Maps for Histograms: Generalized Aitchison Embeddings, Machine Learning Journal, 2014.
T. Le, MC, Generalized Aitchison Embeddings for Histograms, ACML 2013.
K. Shin, MC, T. Kuboyama, Mapping Kernels for Trees, ICML 2011.
MC , J.-P. Vert, A. d'Aspremont, White Functionals for Anomaly Detection in Dynamical Systems, NIPS 2009.
MC, K. Fukumizu, Kernels on Structured Objects Through Nested Histograms, NIPS 2006.
MC, K. Fukumizu, J.-P. Vert, Semigroup Kernels on Measures , JMLR vol.6 (Jul):1169–1198, 2005.
MC, J.-P. Vert, Semigroup Kernels on Finite Sets, NIPS 2004.
MC, J.-P. Vert, The Context-Tree Kernel for Strings, Neural Networks, Volume 18, Issue 8, 2005. 
MC, J.-P. Vert, A Mutual Information Kernel for Sequences, IJCNN 2004.

Working Papers
MC, Positivity and Transportation, arXiv:1209.2655, 2012.
MC, A. Doucet, Autoregressive Kernels for Time Series, arXiv:1101.0673, 2011.
MC, Positive Definite Kernels in Machine Learning, a survey and teaching document (draft), 2010.
MC, Kernels for Measures Defined on the Gram Matrix of their Support, arXiv:0909.0991v1, 2009.
|